A prototype used for stock market performance prediction is showing strong potential for startups across industries to improve efficiency, consistency, and effectiveness of human reviews of textual reports and information.
Text mining, as this is also called, is a laborious task, and many industries depend upon it.
Thanks to a collaboration between a financial services company and Microsoft, a prototype was developed using Natural Language Processing (NLP), Deep Learning, and Convolutional Neural Networking (CNN) that was meant for predicting a company’s stock performance, but that same technology is also being used for much broader purposes.
One of the many ways startups can benefit from text mining is in improving market intelligence.
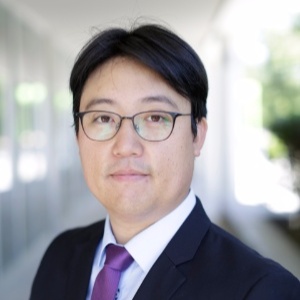
Gene Moo Lee
Gene Moo Lee, assistant professor at The University of British Columbia and former assistant professor at The University of Texas at Arlington, “created a new method that uses big data analytics and text-mining techniques to improve market intelligence and explain potential mergers and acquisitions of startup companies in the fast-moving high-technology industry.”
“Our new approach uses big data analytics and a text-mining technique called topic modeling to identify potential matches,” said Lee, adding, “By analyzing unstructured, publicly available descriptions of any startups’ business, we can quantify any two firms’ business, geographic, investor and social proximity and from there identify potential targets for mergers and acquisitions.”
Referring back to the text mining, stock performance prediction prototype, one of the substantial takeaways was that it was able to predict an under-performing company with up to 62% accuracy, which was almost double what the chance accuracy was at 33.33%.
The team was able to do this “based on the written textual sections of public earnings releases extracted from 10k releases and actual stock market performance,” but it was only through a combined use of NLP, Deep Learning, CNNs, and a whole host of developer tools that made all of this possible.
Startups leveraging text mining
Apart from predicting stock performance or helping startups in market intelligence, there are also many startups that focus on text mining as a major part of their services for a variety of industries.
For example, London-based startup True AI uses text mining to help with customer service by improving customer support in chat services and social media.
Like the Microsoft prototype, True AI uses Deep Learning to suggest possible responses to a user’s queries to which an agent selects and sends a response directly or with edits.
It’s not quite full automation, but again, like the stock performance prototype, it is showing a lot of potential. However, not all businesses are alike. Differences in logistics, resources, access to capital, and of course mission objectives can vary greatly from company to company, so each company must obviously use what is most relevant to its aligned goals.
Another text mining startup, Aylien, uses NLP in a manner that can disrupt many industries including the media, PR, publishing, and marketing through its “article extraction, keyword extraction, classification and sentiment analysis and summarization for most European languages,” as summarized on Medium by TextMining Services OÜ.
Aylien’s Market & Risk Analysis allows users to:
- Track organization mentions.
- Evaluate events and supplier risk.
- Monitor industry and market performance.
- Proactively identify trends and events.
The platform’s Media Monitoring & News Aggregation can benefit PR, startups, and marketers by:
- Evaluating the effectiveness of campaigns
- Tracking brand mentions and shifts in sentiment
- Aggregating content at scale
- Building intelligent news apps and solutions
The benefits of text mining go on and on as what is really at the core of it all is extracting meaningful information from the Himalayas of data.
Where does text mining go from here?
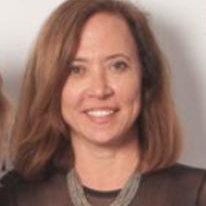
Patty Ryan
Patty Ryan, Principal Data and Applied Scientist at Microsoft, put it quite succinctly on the Microsoft Developer Blog, explaining, “Deep learning models trained on text in earnings releases and other sources could prove a viable mechanism to improve the quality of the information available to those making investment decisions, particularly in avoiding investment losses.”
If the Microsoft team, along with its financial partner, was able to raise a chance accuracy of 33.33% in predicting stock performance up to 62% with its prototype, how far will they be able to take it once its refined, and how disruptive would that be for other industries that follow in their digital footsteps?